Our philosophy
Precision medicine for chronic disease - improving health, for everyone
PrecisionLife’s mission is to find better treatment options for patients with unmet medical needs, particularly in complex chronic diseases.
To find new ways of diagnosing, preventing or treating diseases you first have to understand how they arise and develop, who they will affect and which interventions are going to be effective for each patient.
With complex disorders this requires deep understanding of the architecture of diseases and the stratification of patients suffering from them. This was the promise of the Human Genome Project and every disease project that has collected patient data since, but the limits of existing analysis technologies have often frustrated progress towards better understanding of how to develop better treatment options.
In large part this is due to how we think of diseases – a single diagnostic label, say of Alzheimer’s disease, implies a single cause and, potentially, a single treatment. This is how the blockbuster period of pharmaceutical development was so successful, finding low-hanging fruit where modulation of a specific target provided some help to some patients, and ideally getting this prescribed as the standard of care for all patients with that clinical diagnosis.
We know however that this era is ending – the costs of healthcare has risen, R&D productivity in the pharma industry has fallen and above all, there are still far too many patients with unmet medical needs.
The era of precision medicine is taking over, with the patient at the centre of their health. This patient-centric approach has already transformed oncology, and will inevitably transform the care of much more prevalent and expensive chronic diseases, but to achieve these impacts we will need a radically improved view of these diseases.
Contents
Jump to a chapter by clicking on a link below:
Your guide to precision medicine R&D for chronic disease
Learn how to inform and de-risk every stage of drug discovery and clinical development, to create better treatment options for patients with unmet medical needs.
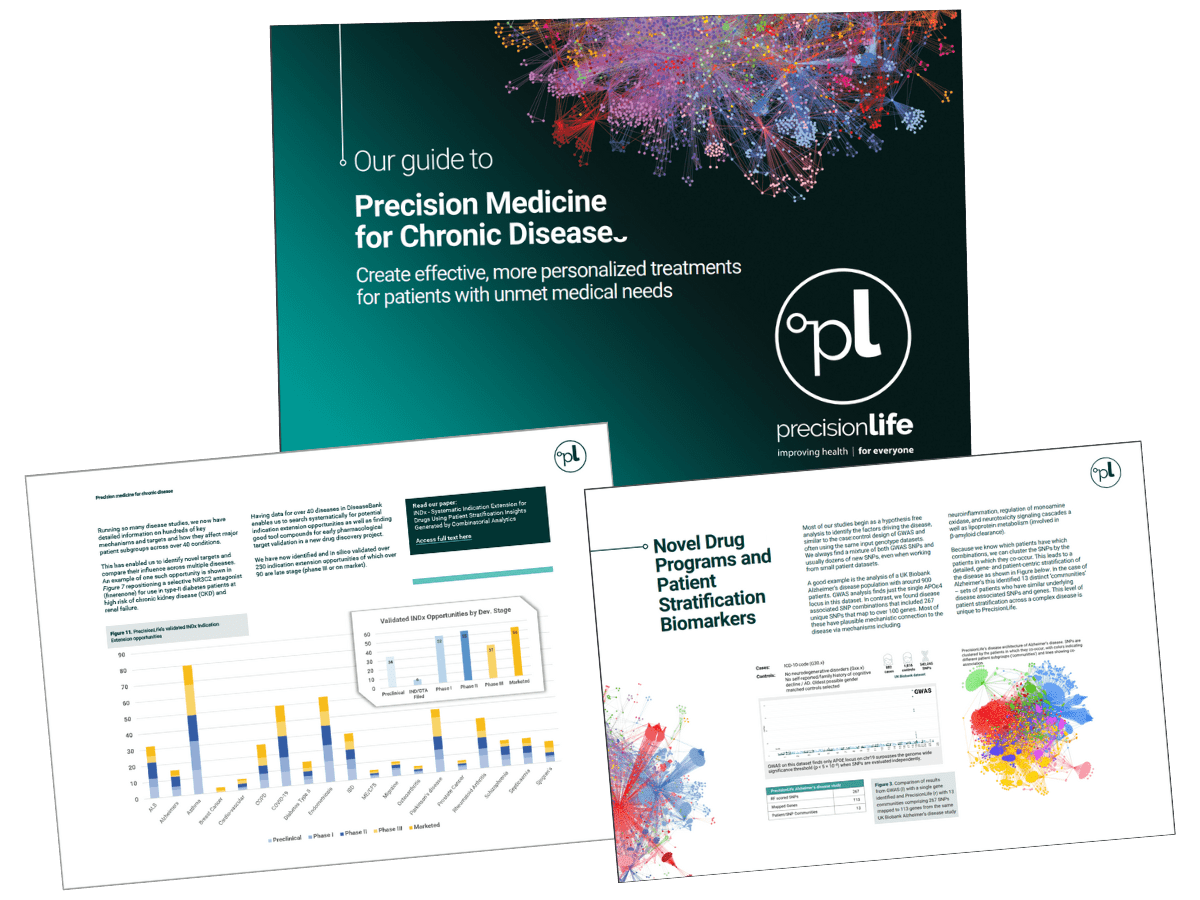
At PrecisionLife we recognized that chronic diseases such as diabetes, dementia, cardiovascular, autoimmune, respiratory, and psychiatric disorders do not arise in the same way as cancer or rare disease, and that different tools will be needed to study them.
We believe that chronic diseases don’t arise simply from mutations in single genes. They’re caused by a complex interplay of multiple genes and other factors – technically they’re polygenic and heterogenous. Their complexity means that patients with the same diagnosis may well have different mechanistic etiologies, and will benefit from different therapies based on their personal make-up and circumstances. These differences don’t show up in the current gold-standard genomic analyses such as GWAS studies, and genomic medicine has therefore had limited impact on these diseases.
We developed our platform specifically to tackle chronic diseases, enabling us to identify and understand more deeply the biological mechanisms that are driving disease within subgroups of patients.
We’ve developed innovative ways to analyze disease population datasets and mechanistically stratify different patient subgroups. We use the insights we generate not only to find better new ways of treating that disease, but also to improve the diagnosis and inform the selection of the most effective existing medicines for individual patients.
We’re taking four complementary approaches, all driven by deep understanding of the biological drivers of disease in patient subgroups:
- Finding novel drug targets and leads for unmet medical needs
- Finding opportunities to use existing drugs to treat additional diseases
- Finding ways to predict and prevent chronic disease
- Accurately diagnosing individual patients and choosing the most effective medicines for them
The first two approaches are primarily relevant to biopharma companies, and the last two to healthcare organizations, but there is important overlap, and they are all based on the same deep disease insights that we have generated for 50 diseases with the PrecisionLife platform.
2. Our Approach to Complex, Chronic Diseases
Chronic diseases (e.g., dementia, diabetes, cardiovascular, respiratory and autoimmune disorders) cause suffering for billions of patients and cost trillions of dollars to treat every year. They account for over 80% of healthcare spending but haven’t been impacted by genomic medicine as much as cancer or rare diseases. The limitations of these studies was well described in Tam et al. 2019[1] and summarized in Figure 1 below.
Our concept of chronic disease is different to traditional genomic approaches, and our combinatorial approach addresses all of the weaknesses of the current GWAS based tools.
Rather than looking for more and more ultra-rare variants in single genes to explain disease, we analyze how interactions between multiple relatively common variants and other external factors come together to trigger disease processes. In many cases some of the genetic factors occur in expression control regions, outside of coding regions for genes, making whole genome or even genotyping approaches more useful than whole exome sequences.
Finding the SNPs and other features whose non-linear interactions have measurable impact on a clinical phenotype allows us to perform high-resolution patient stratification. From this we can explain how the various aspects of disease biology affect different subgroups of patients and identify novel drug targets relevant to those patients.
The key challenge for biopharma and healthcare is to understand which targets and drugs will work with which subgroups of patients. Understanding this uncovers new disease biology and drug targets, but it also accelerates and derisks the clinical development of new drugs, creates drug repositioning opportunities, and leads to better diagnosis and prescription of more effective therapies for patients. We have created an Innovation Engine to deliver these insights across dozens of chronic diseases.
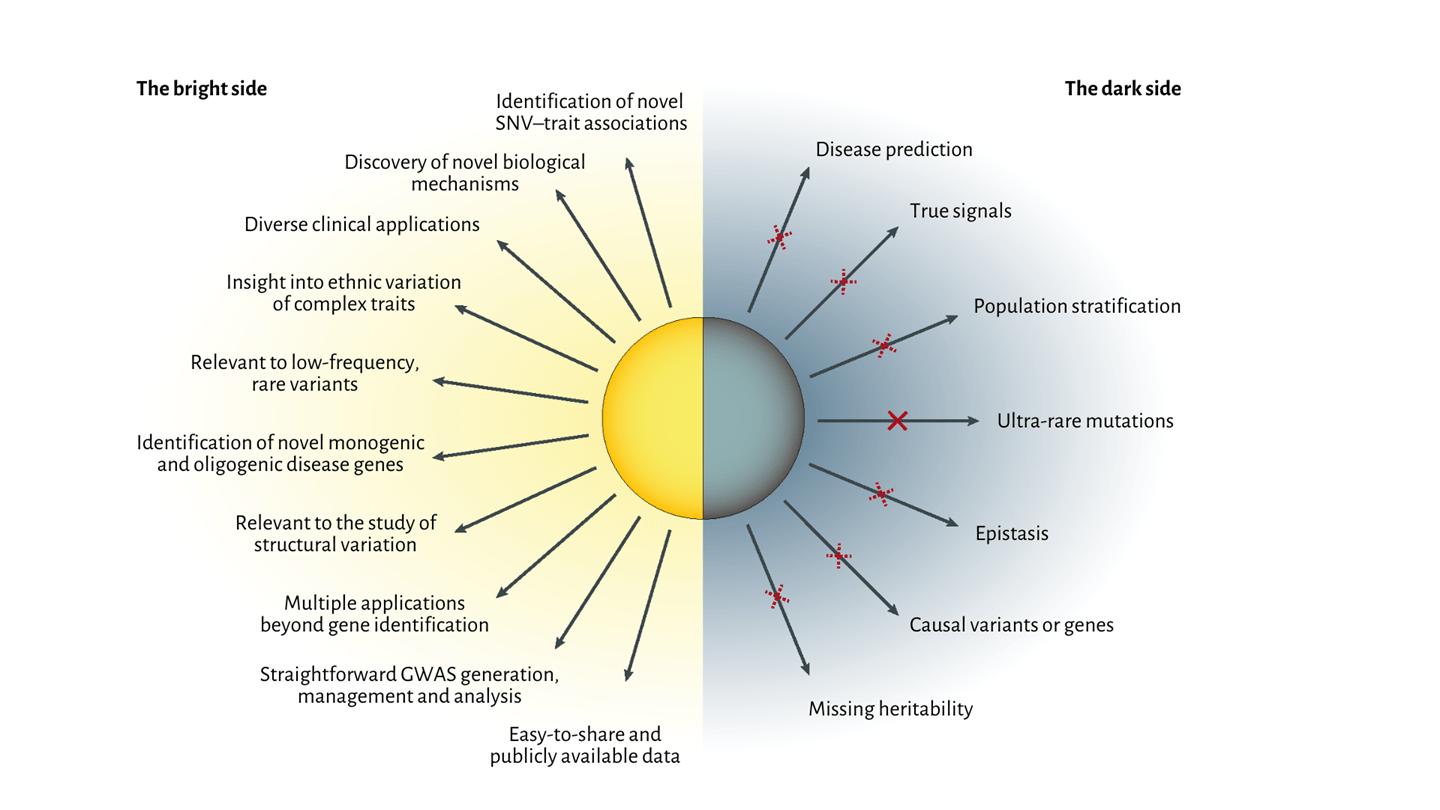
Figure 1. Benefits and limitations of GWAS approaches from Tam et al. 2019.
3.The Benefits of Combinatorial Analytics vs GWAS
GWAS are designed to find single SNPs that occur more often in a patient population (cases) than in controls. GWAS assume the effects on disease of those SNPs are independent, additive, and the same across all patients. It ignores patient subgroups and all of the interactions between SNPs/genes. It focuses more on adding rare variants to explain more of the disease, but because these are often specific to particular populations, disease models built on them often don’t translate well between different ethnic backgrounds.
One of the huge challenges in GWAS is the need to remove linkage disequilibrium (LD) effects, where SNPs that are located closely on a chromosome may be over-represented in results only because of their proximity to a disease-causing locus rather than any causal effect. LD clumping basically chooses a single SNP (with the lowest p-value) to represent the effect of all of the closely located SNPs (usually tens of thousands).
The problem is that important non-linear effects do exist between SNPs that are in LD with each other[2]. Decades of structural biology and gene expression control insights clearly show that different mutations in very closely located bases, even in the same base pair, can have markedly different impacts on phenotype. In some interesting extreme cases one genotype may cause disease while another genotype at the same SNP locus may protect from disease[3]. GWAS can’t distinguish these, but when we use combinatorial analysis, we can see that in these cases the biological context of these effects – their interactions with other genes – is different.
GWAS is therefore an inherently low-resolution technique that misses much of the real disease signal in highly polygenic diseases. Because its signals are fundamentally based on population averages, it cannot readily stratify patients at a useful level of detail. Machine learning and polygenic risk scores (PRS) built on the results of GWAS cannot reconstruct these critical non-linear interactions and so are also incomplete and lack predictive power in chronic diseases, especially across populations with different origins[4].
To address this PrecisionLife has developed a unique combinatorial analytics approach that identifies interactions between multiple relatively common variants and other clinical and epidemiological factors that together have an impact on the patient’s phenotype. The combinations that we identify enable us to build a much more detailed picture of the complex, interconnected biology of these chronic disease populations. This captures the different mechanistic origins of disease biology and how these affect different subgroups of patients, ultimately helping identify novel drug targets relevant to those patients.
Figure 2. PrecisionLife combinatorial disease signature comprising 6 co-associated SNPs.
4. Disease Studies and DiseaseBank™
At PrecisionLife our approach to disease studies is data driven and usually hypothesis free with no need for training models. We use an iterative approach starting from simple single feature associations, finding and validating new features to add to these to build ever more complex combinations of features.
Our studies use multi-modal patient datasets which might include genomic, transcriptomic, clinical, epidemiological and even imaging and wearables data. The sources of these data range from national biobanks, research consortia and disease charities to pharma companies’ clinical trials datasets and disease specific populations we have commissioned with partners.
We’ve pioneered a new combinatorial analysis approach that reveals many more of the biological drivers of disease than GWAS or related methods. Uniquely these include the effects of non-linear interactions between genes and other epidemiological and environmental factors. The much deeper disease insights we generate allow us to better stratify patients according to the mechanistic origins of their disease.
Our studies can be constructed in different way to answer different questions:
- Finding causative disease risk / protective factors and novel druggable targets
- Stratifying patient populations and finding mechanistic biomarkers
- Comparing the drivers of disease in two patient cohorts, e.g. T2 and non-T2 asthma
- Understanding differences leading to a quantitative outcome, e.g., drug response vs non-response in clinical trials:
We’ve run our combinatorial analysis platform across 50 chronic disease datasets (and we’re adding 2 per month). Our studies have generated deep insights into the mechanisms underpinning all of these diseases, far surpassing the results that can be generated by GWAS on the same datasets.
For each of these complex chronic diseases, we’ve discovered new biomarkers that identify all of the clinically relevant patient subgroups who share a disease mechanism and found novel drug targets and indication extension opportunities for treating them. All these proprietary and protectable insights are stored in our DiseaseBank repository.
5. Novel Drug Programs and Patient Stratification Biomarkers
Most of our studies begin as a hypothesis free analysis to identify the factors driving the disease, similar to the case:control design of GWAS and often using the same input genotype datasets. We always find a mixture of both GWAS SNPs and usually dozens of new SNPs, even when working from small patient datasets.
A good example is the analysis of a UK Biobank Alzheimer’s disease population with around 900 patients. GWAS analysis finds just the single APOε4 locus in this dataset. In contrast, we found disease associated SNP combinations that included 267 unique SNPs that map to over 100 genes. Most of these have plausible mechanistic connection to the disease via mechanisms including neuroinflammation, regulation of monoamine oxidase, and neurotoxicity signalling cascades as well as lipoprotein metabolism (involved in β-amyloid clearance).
Because we know which patients have which combinations, we can cluster the SNPs by the patients in which they co-occur. This leads to a detailed, gene- and patient-centric stratification of the disease as shown in Figure 3. In the case of Alzheimer’s this identified 13 distinct ‘communities’ – sets of patients who have similar underlying disease associated SNPs and genes. This level of patient stratification across a complex disease is unique to PrecisionLife.
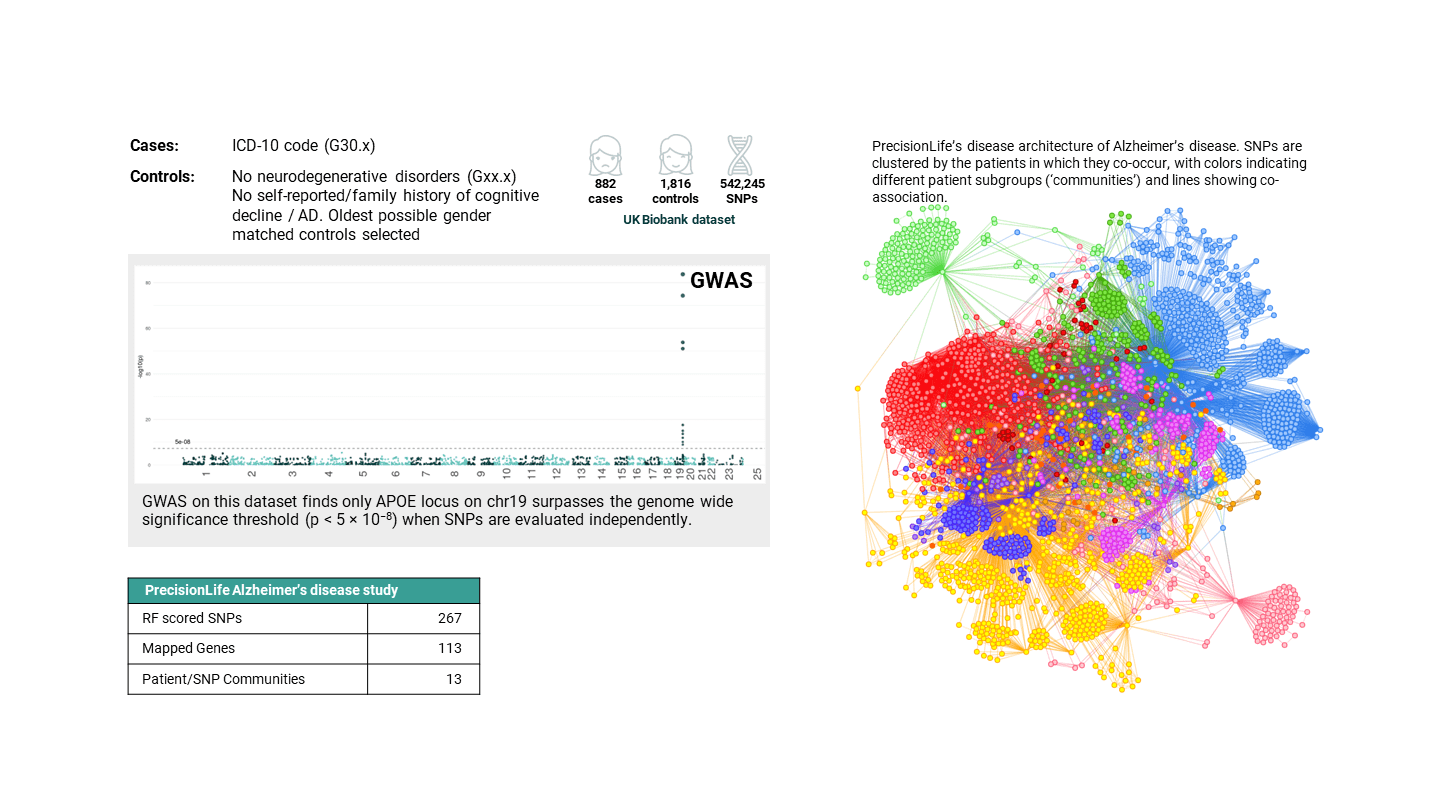
Figure 3. Comparison of results from GWAS (left) with a single gene identified and PrecisionLife (right) with 13 communities comprising 267 SNPs mapped to 113 genes from the same UK Biobank Alzheimer’s disease study.
By comparing the pathways of these genes we can further stratify the patient population into 6 subgroups, each with a distinct mechanistic basis driving their form of the disease. These range from lipoprotein metabolism to changes in immune function caused by prior viral infection through metabolic syndrome and dysregulated neurotransmitters.
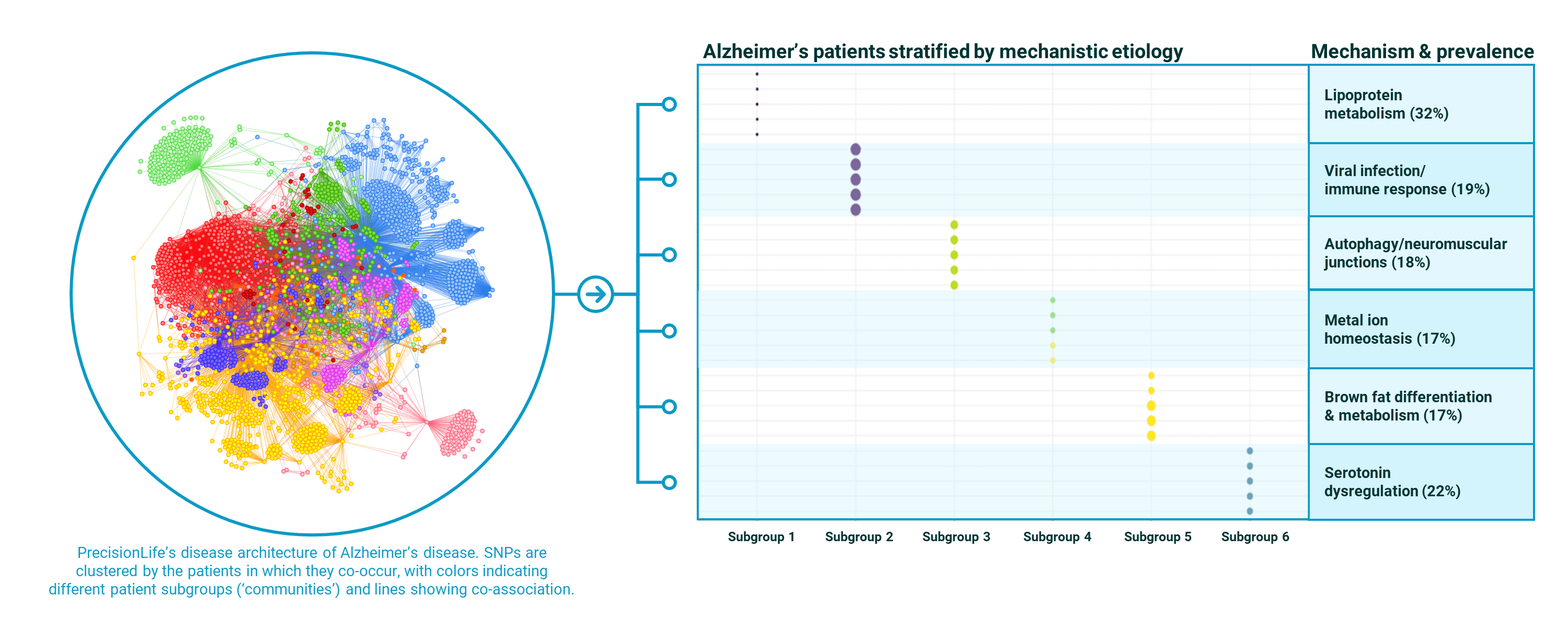
Figure 4. PrecisionLife stratification of a UK Biobank Alzheimer’s disease population into 6 mechanistically distinct patient subgroups with case prevalences for each mechanism in the Alzheimer’s population shown.
It can be seen that the penetrance of these different mechanistic drivers of Alzheimer’s is only between 17%-32%. This sets an upper limit on the degree of clinical efficacy it will be possible to demonstrate for a drug targeting that mechanism if patients are chosen at random from those with an Alzheimer’s diagnosis.
As 32% response is not sufficient to demonstrate clinical efficacy, without a complementary patient stratification biomarker this goes some way to explaining the 100+ failures in clinical trials for drugs targeting lipoprotein metabolism and its effects on β-amyloid clearance.
The analysis also holds promise for new treatments – there are new druggable targets identified in each of the mechanisms with patient stratification biomarkers to delineate potential responders. There is also the opportunity for combination therapies or polypharmacology as over 70% of patients have features from more than one of the disease mechanisms in their make-up.
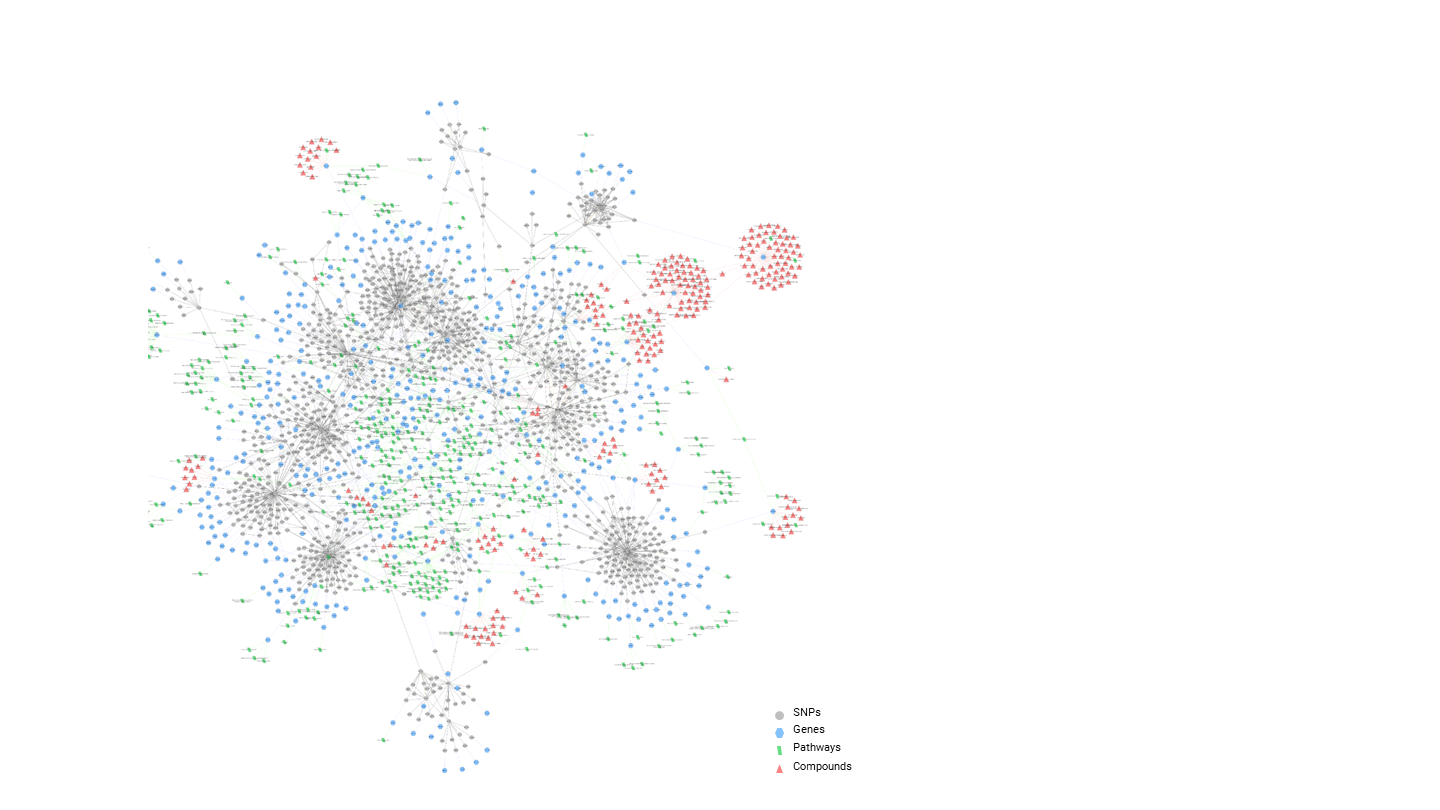
Figure 5. PrecisionLife’s stratification of an ALS population showing major patient subgroups and the SNPs, genes, pathways and known active chemistry available for each of the novel targets identified.
For rare disease like ALS we have also performed the first detailed genetic stratification of the disease, identifying multiple clusters of patients sharing common genetic and exogenous factors, epidemiology and secondary diagnosis. This creates opportunities to treat different populations exhibiting different presentations of the disease. We have identified multiple novel targets and associated lead compounds that demonstrated potential disease modifying activity, some of which are being developed with a view to moving quickly to first-in-man studies.
In our novel drug discovery programs, the disease insights are used as input to a detailed triage process. This starts in silico with the annotation of SNPs, genes and other features using a knowledge graph from over 50 data sources. This allows 5Rs type criteria to be applied to the genes to identify their tractability from a druggability perspective. Similar criteria can be applied regardless of modality, and we explicitly search for opportunity to target cell surface receptors with monoclonals or transcription factors with siRNAs, as well as traditional small molecule modulators of target activity.
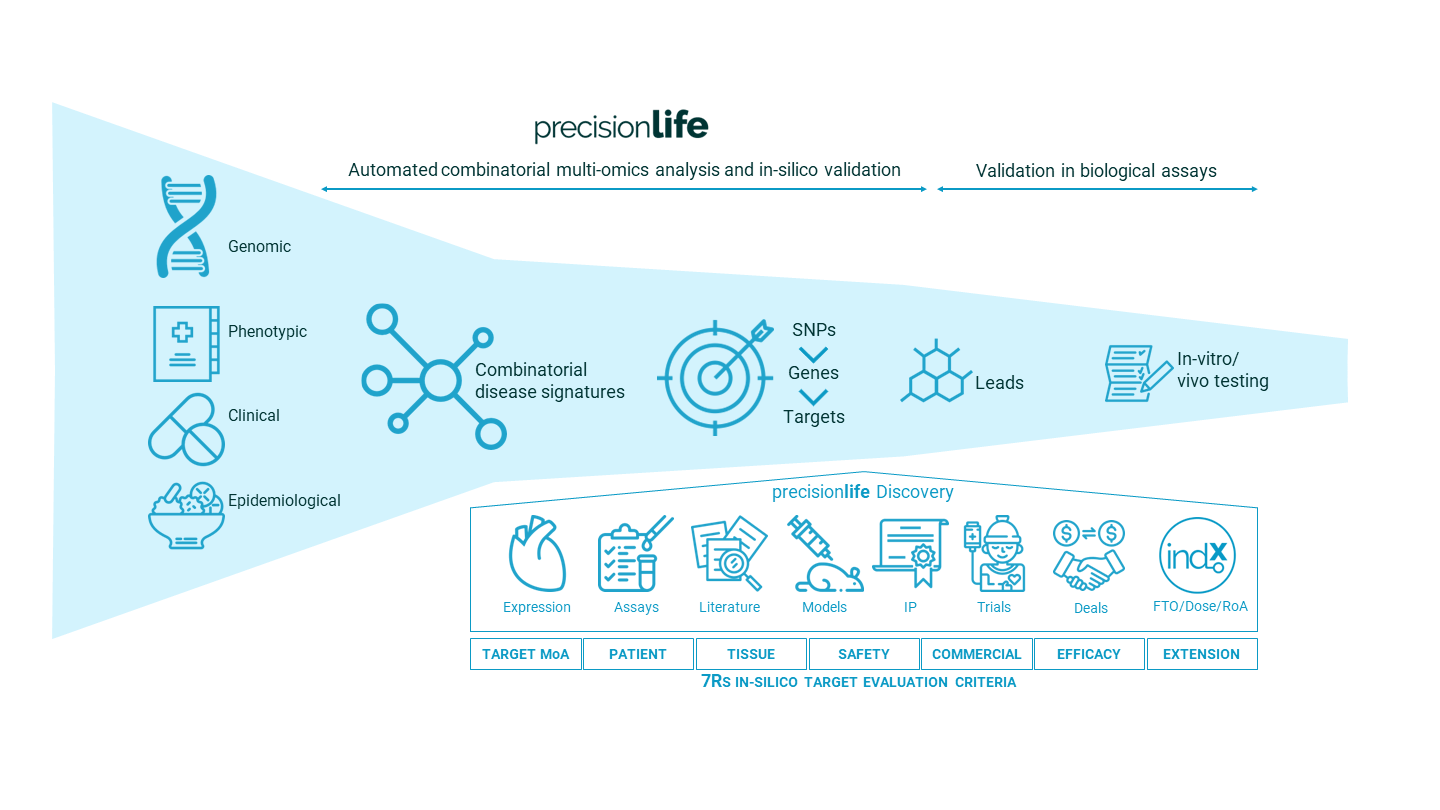
Figure 6. PrecisionLife’s novel drug program discovery & validation process.
This enables a new range of highly innovative targets to be evaluated, with PrecisionLife’s supporting Efficacy Score metrics. These show the potential to use the biomarkers identified to clearly stratify a potential responder population to accelerate trials and de-risk downstream clinical development.
6. Clinical Trial Enrichment with Mechanism-based Patient Selection
Patient stratification and the use of biomarkers to identify patients who are likely to respond to specific drugs is essential to derisking clinical development projects and can also be used to support the product launch of a new drug.
There are 3 main ways in which such patient stratification biomarkers (PSBs) can be used to enrich clinical trials:
1. Prospectively
Using mechanism specific PSBs as inclusion criteria for recruiting likely responders into a clinical trial population - enriching clinical trials to increase their probability of success
2. Reactively
Developing PSBs to support a target or target product profile (TPP) that has already been selected and validated to make further clinical development faster, cheaper, and more likely to be successful
3. Retrospectively
Analyzing the drug response of patients in Phase III clinical trials to develop novel biomarkers of drug response that can be used in a regulatory submission and/or clinical settings
Mechanism-based Patient Stratification Biomarkers for Prospective and Reactive Trial Enrichment
PrecisionLife’s patient stratification analysis automatically classifies patients into different subgroups based on the mechanistic etiology underpinning their form of the disease. As well as directly implicating specific targets in the disease, these also serve to indicate the broader mechanisms that are affected and therefore the drugs that might modify disease in that subgroup of patients.
The prevalence and clinical relevance of the mechanism is important for the quantifying the likely level of clinical efficacy that can be demonstrated by a drug targeting a specific gene. In the case of APOε4, this appears to be only about 1/3 of the UK Biobank population, which is a relatively early-onset cohort. Without choosing patients with this specific disease signature, 32% is the maximum efficacy that is likely to be demonstrated in a clinical trial, even for a highly effective drug.
The phase III trials that fail unnecessarily potentially waste hundreds of millions of dollars of development costs and billions in uncaptured revenues, and they prevent effective medicines from reaching patients with unmet medical needs. Designing studies with mechanism based PSBs improves the chances of avoiding costly late-stage efficacy failures and provides a rationale to engage with regulators to design smaller, faster to read out clinical trials.
It may also open the opportunity to design evidence-led adaptive trials around the specific needs of patients, perhaps even extending into PSB led combination therapies for some patients with particularly clear biomarkers signatures.
The PSBs identified by PrecisionLife may not simply be diagnostic – they may also predict specific phenotypes, for example early-onset of disease or fast progression rates, as in the case of ALS. Figure 7 shows the stratification of a UK ALS cohort into 3 distinct subgroups with different age and site of onset, progression rates, sex split and secondary diagnosis. Cluster A is a group of the fastest progressing patients as shown by their age at death and survival statistics.
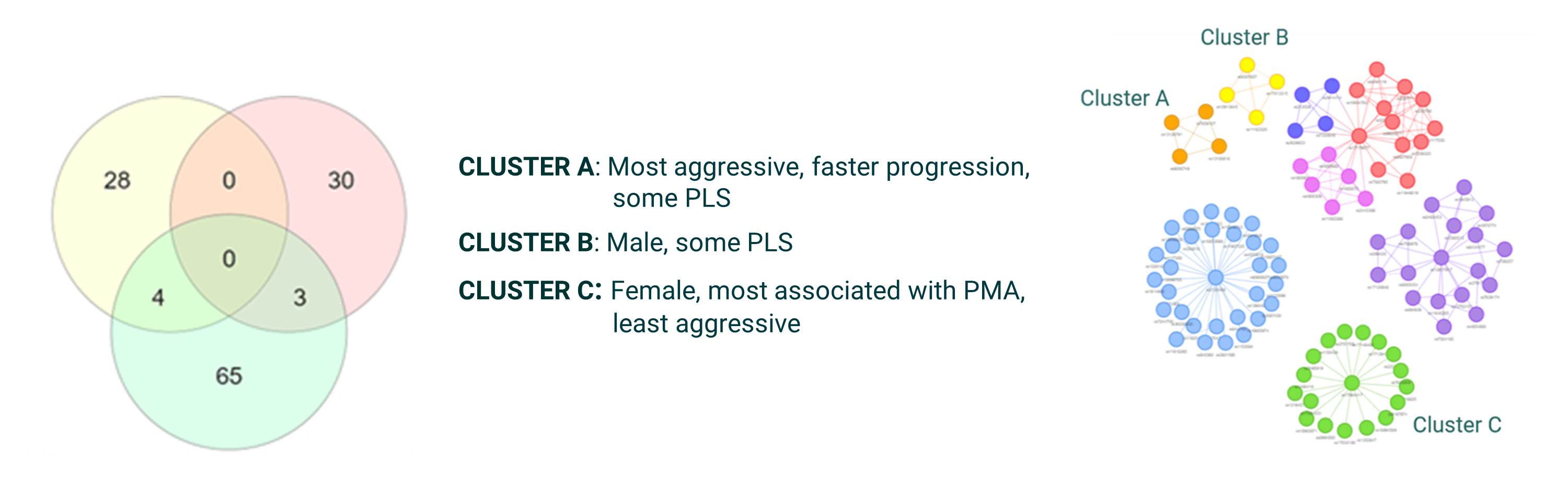
Figure 7. PrecisionLife stratification of a UK ALS population revealed 3 distinct subgroups with different clinical presentations, progression rates and genetic associations.
The PSBs may even predict secondary effects such as renal, ophthalmic, or cardiovascular complications of type-II diabetes. An example is shown below, identifying type-II diabetes patients at risk of chronic kidney disease whose symptoms are likely to be ameliorated using selective mineralocorticoid receptor antagonists such as finerenone. Understanding secondary prevention options for patients is a major untapped opportunity.
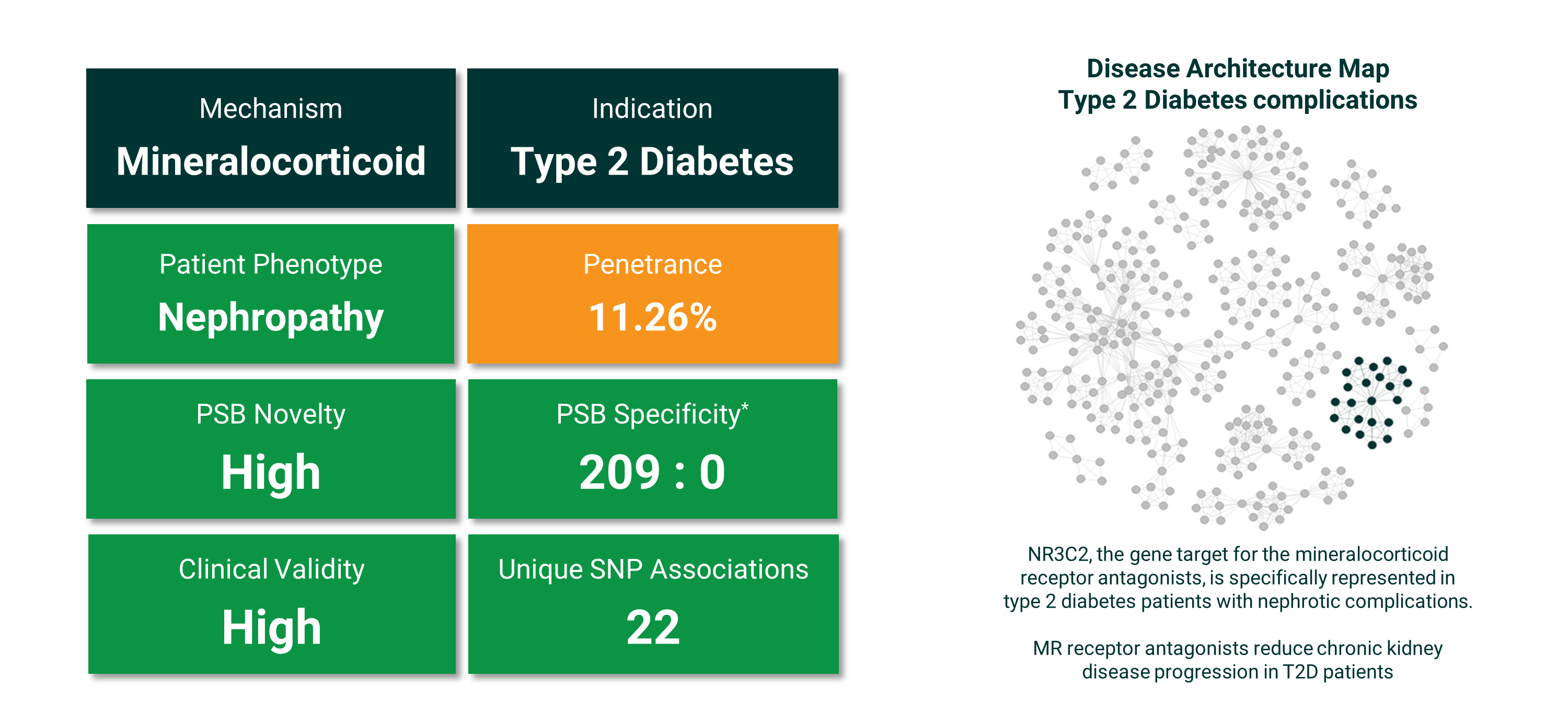
Figure 8. PrecisionLife stratification of a UK Biobank type-II diabetes complications population into 5 distinct complications subgroups with the case prevalence and PSB specificity shown for patients at high risk of renal failure and dialysis. This represents around 1 in 9 patients and the PSB is a very specific (209 cases : 0 controls) combination of 22 SNPs. Right: the highlighted group of SNPs (dark) contains the variant in NR3C2 that was associated with patients who developed renal complications.
Genetic biomarkers can be very useful for evaluating a patient’s risk of developing a disease or categorizing their likely progression rate or response to a therapy, but they may not directly indicate a diagnosis of the disease or its stage of development.
If the gene product is not directly diagnostic, understanding the mechanistic driver of disease from the metabolic context provided by the PrecisionLife disease signatures enables the identification of other more diagnostic biomarkers, which might include metabolites from blood, saliva or combinatorial profiles of analytes from liquid biopsies. These can be particularly useful in the context of early diagnosis of degenerative conditions such as Alzheimer’s, ALS, and ME/CFS where there are no biomarkers with good correlation to clinical outcomes.
PSBs may not be solely (or even at all) genetic of course – they may be made up of combinations of genetic and non-genetic features (such as test results, epidemiological and/or clinical features).
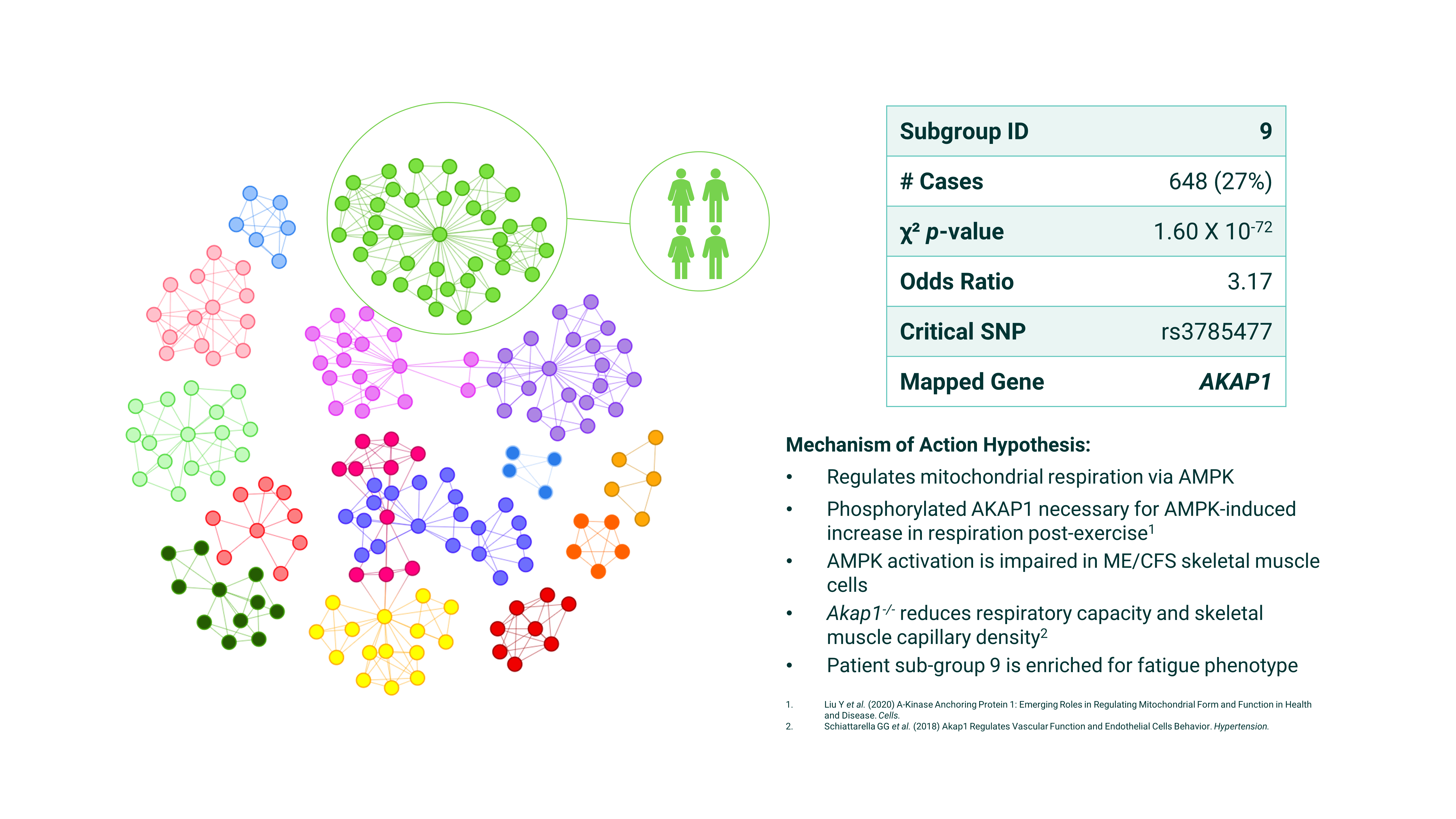
Figure 9. PrecisionLife’s ME/CFS disease architecture - SNPs are clustered by the patients in which they co-occur, with colors indicating different patient subgroups and lines showing co-association.
Drug Response Biomarkers for Retrospective Trial Enrichment
Patient stratification biomarkers are not only useful prospectively as inclusion criteria to design better targeted clinical trials containing more responders, which can be smaller and faster to read out. They can also be generated from retrospective analysis of Phase III clinical trials to identify the features that differentiate patient who show a strong drug response.
Many late-stage clinical trials failures are due to an inability to demonstrate clinical efficacy, incurring huge expense, disruption and impact on company value. This may happen when the chosen drug target’s biology is imperfectly mapped to the actual underlying disease mechanisms for the patients who have been enrolled in the clinical trial. This means that the drug simply won’t work for a majority of patients.
By analyzing the level of drug response as a quantitative trait, PrecisionLife is able to identify the combination of features associated with strong drug responders. These drug response biomarkers can be used with regulators, to refine clinical trial design, and also as complementary diagnostic tools to support new therapeutic product launches.
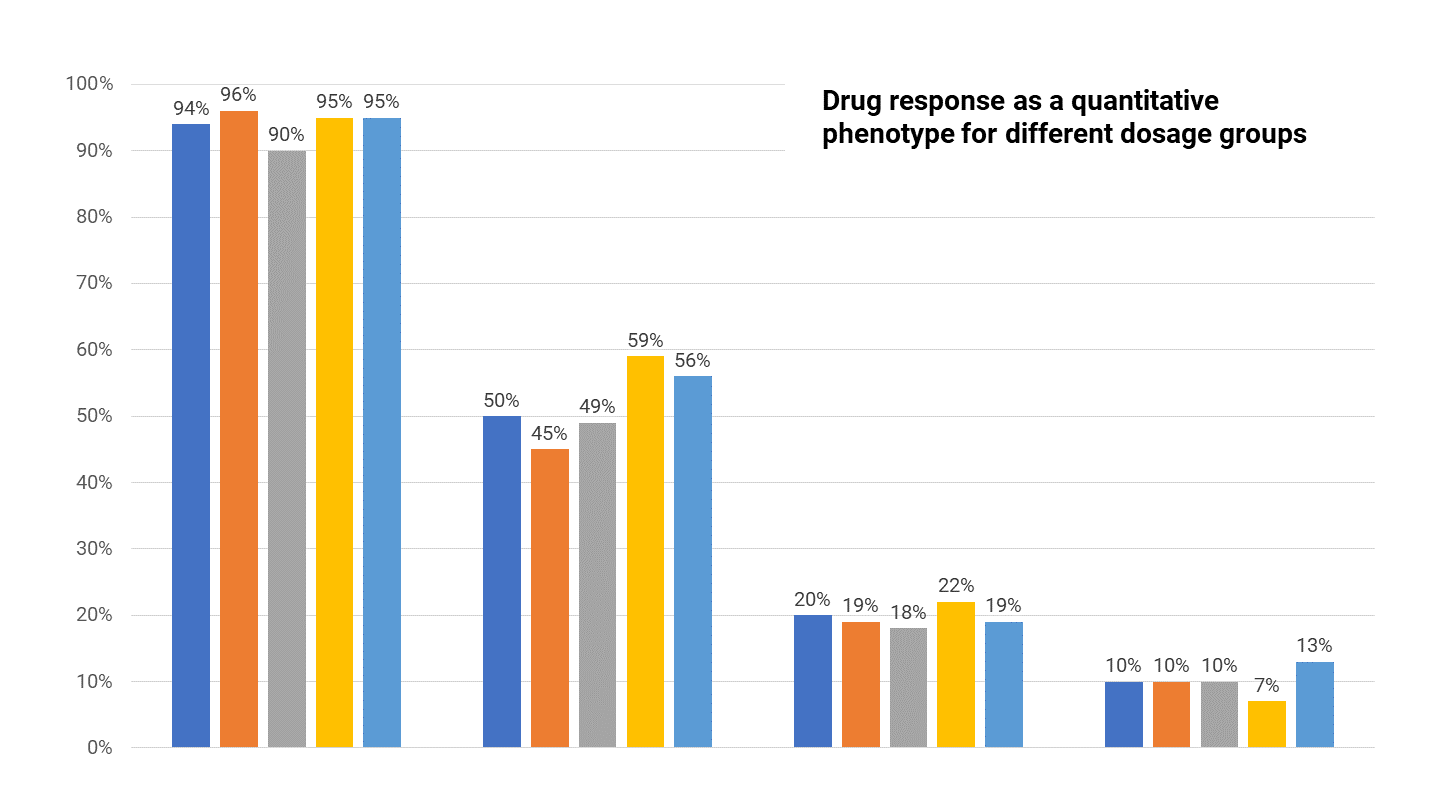
Figure 10. Clinical trials drug response rates across patients given different doses of a drug.
7. Identifying Indication Extension Repositioning Opportunities
It is early days for novel drug programs that have to go through the full clinical development process, but progress can be made quicker with precision repositioning of existing drugs and development candidates in secondary indications guided by patient stratification biomarkers that delineate the patient subgroups whose disease would benefit from a drug targeting that specific mechanism.
Indication extension or repositioning of drugs can, if done well, provide a faster, cheaper and derisked route to the approval of new therapies, creating new options to address pockets of unmet medical need for patients and offering the potential for significant commercial and clinical benefits.
While offering potential speed, cost and risk benefits, repositioning still faces many of the same challenges as novel drug discovery – hypothesis generation, understanding the mechanism of action(s), identifying potential responders and establishing a robust patent position. Additionally, drug safety and toxicity assessment, along with an evaluation of the dosage and route of administration, is needed to identify any potential safety concerns associated with the drug and new indication(s).
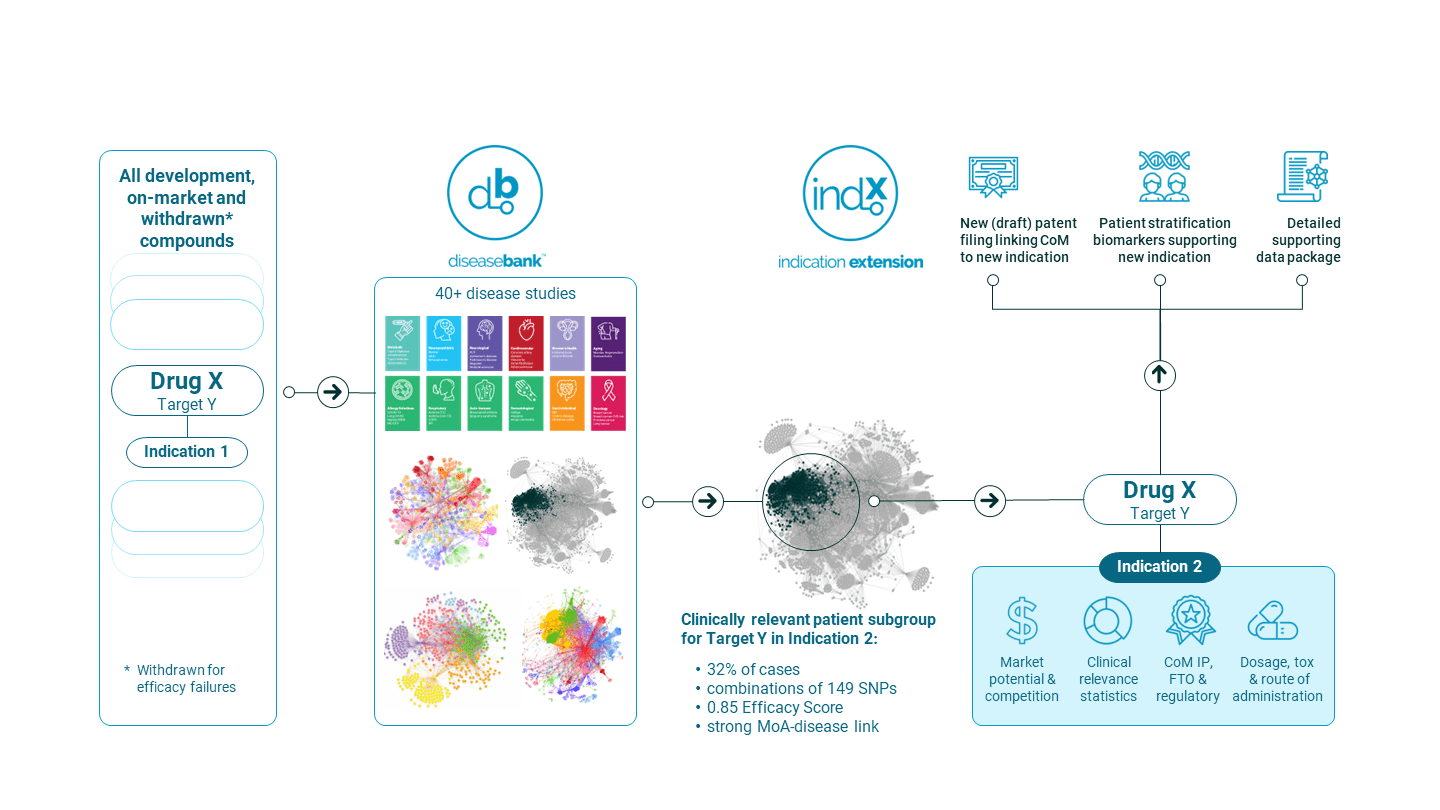
Figure 11. PrecisionLife’s INDx Indication Extension discovery & validation process.
We now have detailed information on hundreds of key mechanisms and targets and how they affect major patient subgroups across multiple conditions. This has enabled us to identify novel targets and compare their influence across diseases. An example of one such opportunity is shown in Figure 8 , repositioning a selective NR3C2 antagonist (finerenone) for use in type-II diabetes patients at high risk of CKD and renal failure.
Having data for over 50 diseases in DiseaseBank enables us to search systematically for potential indication extension opportunities as well as finding good tool compounds for early pharmacological target validation in a new drug discovery project. We have now identified and in silico validated over 250 indication extension opportunities of which over 90 are late stage (phase III or on market).
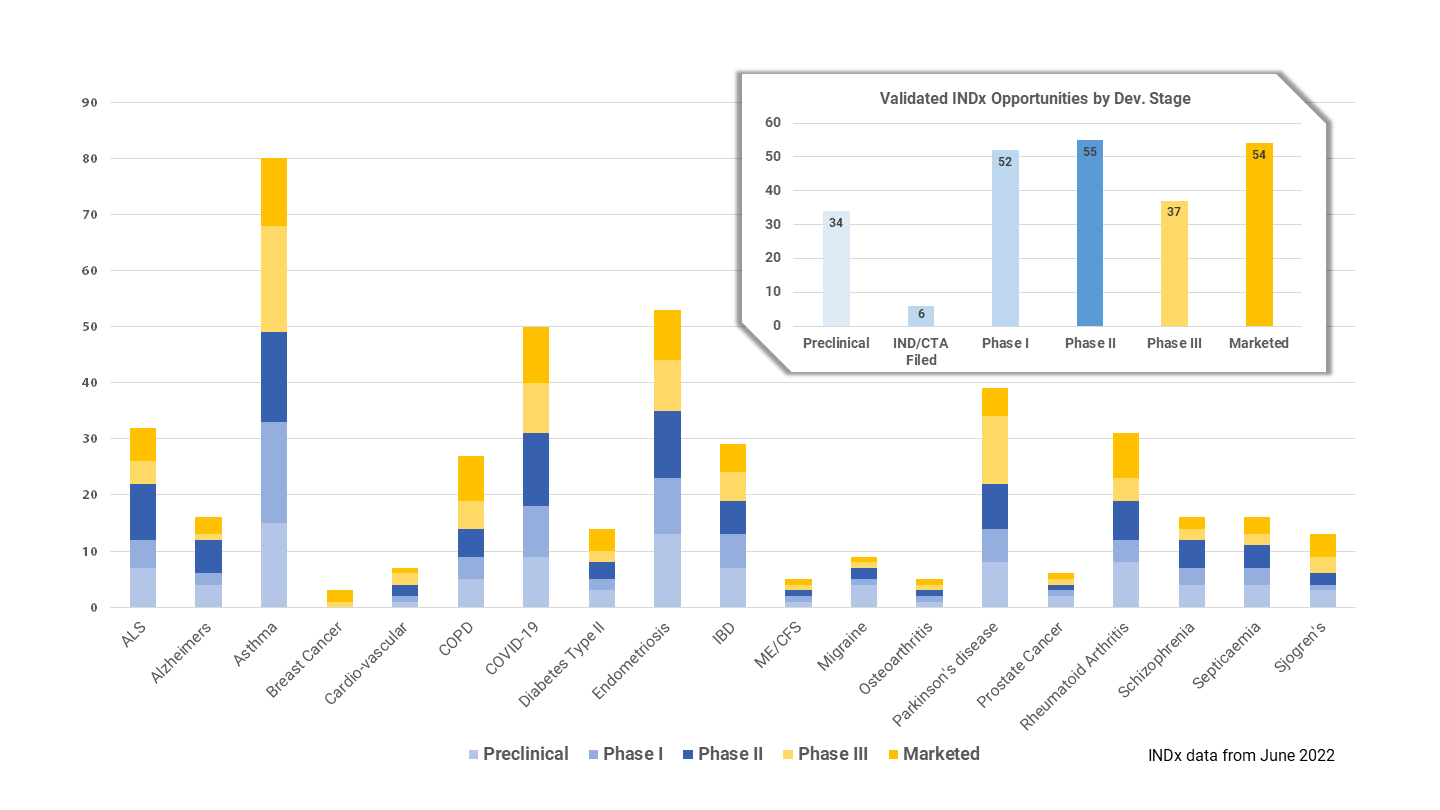
Figure 12. PrecisionLife’s validated INDx Indication Extension opportunities.
The insights contained in DiseaseBank form the data packages necessary to support IP filings for a range of purposes. These include method of use patents linking a drug to a new indication supported by responder biomarkers, patient stratification biomarkers as novel diagnostic tools, and proprietary risk models and clinical decision support tools.
8. Prediction & Prevention of Chronic Diseases
At PrecisionLife we’re exploiting our unique understanding of the factors driving disease for different patient subgroups to build a new generation of precision medicine tools. We believe that the best way to mitigate the personal, social and economic impact of chronic diseases is to help patients avoid their worst consequences for as long as possible. Prediction and prevention via earlier, better targeted interventions help to minimize the development of the most serious symptoms of chronic diseases.
Our combinatorial risk scores predict more accurately the correct diagnosis for an individual patient and help identify their most effective treatment options. We are extending the reach of precision medicine outside of cancer and rare disease into a wide range of complex chronic diseases, working with partners to deliver:
- More accurate and personalized diagnostic / prognostic tools
- Better prediction and prevention of individual patient’s risk in chronic diseases
- Guidance to help select the most effective treatment options in complex patients
Precision medicine is based on understanding a patient’s individual risks and likely response to therapeutic and non-therapeutic interventions. This is an area where GWAS and PRS tools built on them have had great success, transforming care in monogenic diseases such as cancer. However, these tools suffer from limitations and biases that mean they don’t work as well in highly complex polygenic disorders.
Polygenic risk scores (PRS) assume that SNP contributions are independent of each other, work the same in every patient, and can be added together. None of those assumptions work well in polygenic diseases. Chasing ever rarer variants in often biased founder populations to add more SNPs with infinitesimal power to a PRS deprives the models of explanatory value and makes them less transferrable between populations with different backgrounds.
In contrast PrecisionLife’s combinatorial risk scores (CRS) take full advantage of the non-linear impacts of combinations of genetic, clinical and epidemiological features and the high-resolution patient stratification showing different disease etiologies for different patient subgroups. By understanding the impacts of all the factors driving disease in a patient, their personal risks, prognoses and likely therapy response can be more accurately predicted.
PrecisionLife’s CRS can accurately assess the personal risk level and factors for a patient:
- Keeping patients healthier for longer and avoid development of the worst symptoms
- Giving a more accurate and informed diagnosis
- Providing insights into the underlying causative mechanisms for disease in a patient
- Allowing targeting of resources towards patients at risk of the most serious forms of disease
- Helping select the most effective treatment options for an individual patients based on their profile
A good example is the complications associated with type-II diabetes. Diabetes is one of the most significant global health and economic burdens across both developed and developing countries, costing over almost $2 trillion worldwide and $450 billion in the US alone.
Complications associated with type 2 diabetes (including dialysis, strokes, blindness and amputations) account for 80% of this spending, due to expensive additional hospitalizations and treatments. They cause poor quality of life for patients, leading to higher long-term social care costs and mortality rates. Complications of diabetes also predispose patients to long-term debilitating conditions such as cardiovascular disease and various forms of dementia.
To identify at-risk patients early and intervene effectively we need to be able to predict, at the point of diagnosis, an individual’s predisposition to a specific disease outcome. We built a diabetes complication disease model by analyzing a dataset from the UK Biobank, comparing 2,900 diabetics with complications against 5,800 of the oldest gender & BMI-matched diabetic controls who had not (yet) developed any complications. Our analysis targeted renal failure, cardiovascular events, ulcer/amputation, neuropathy, and blindness complications.
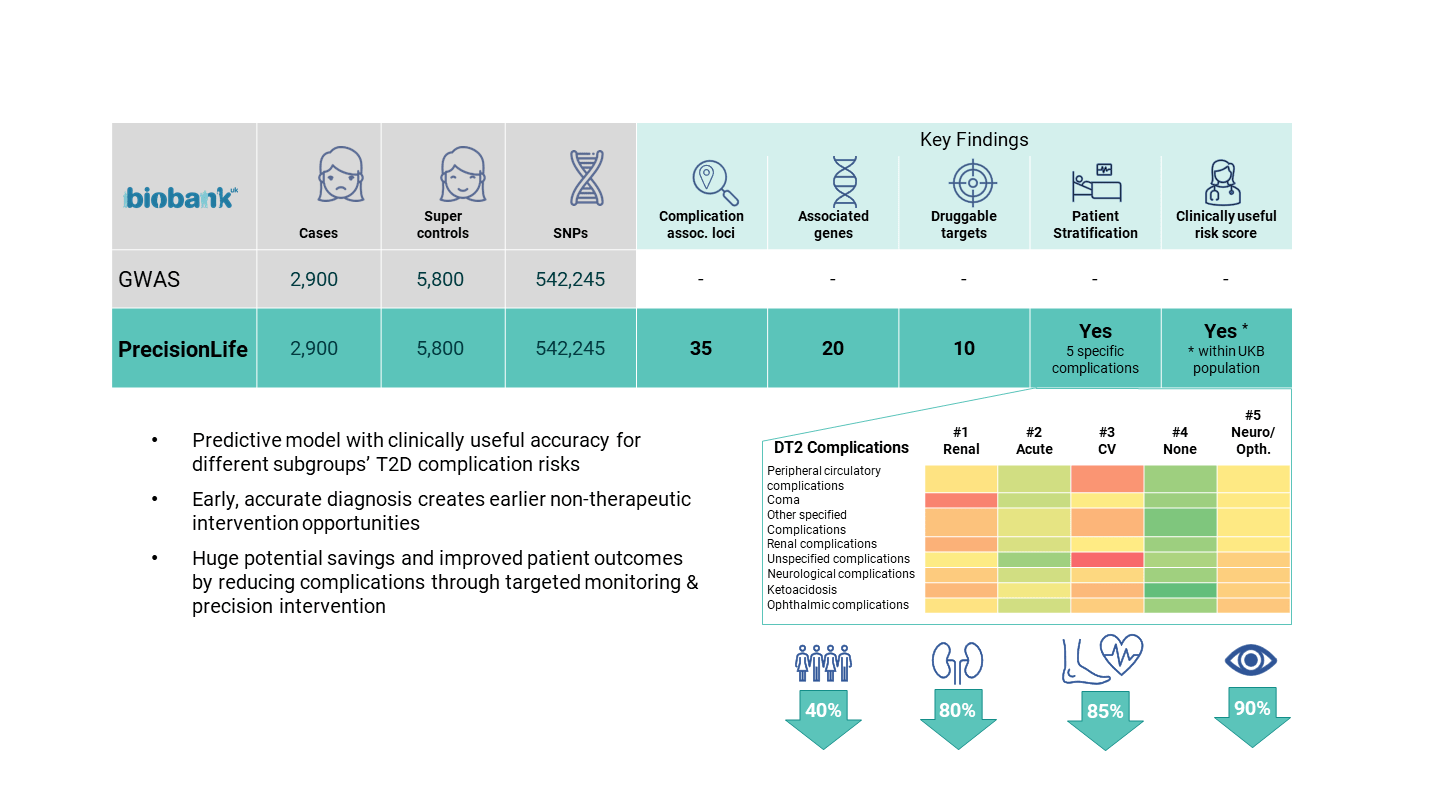
Figure 13. PrecisionLife’s type-II diabetes combinatorial risk score model.
We found significant genetic differences between the patients with and without diabetes complications, indicating that there is a subset of diabetic patients who are predisposed to severe diabetes and diabetes-related complications, independent of lifestyle and environmental factors. We then built a diabetes complications CRS model that was able to predict the disease trajectory for individual patients across the 5 main types of complication.
The personalized intervention recommendations suited to each patient subgroup can be determined by a clinical decision support tool (CDS) built on the disease insights and the patient’s personal make-up and then delivered to their clinician for their consideration.
9. Better Diagnostics and Clinical Decision Support
The ultimate goal of precision medicine is to enable prescription of the right drugs to the right patient at the right time. PrecisionLife’s insights into complex chronic diseases are powering a range of diagnostic, prognostic and therapy selection tools that identify the best interventions to preserve the patient’s quality of life.
Because of their inherent complexity, patients with a chronic disease may share the same diagnosis, but this doesn’t mean the causes of their disease are identical. There are multiple biological mechanisms that can go wrong that will lead to the same clinical symptoms. This means that different drugs will be more successful in some subgroups of patients than others, depending on which mechanism they target.
A good example is asthma. Asthma patients can be broadly categorized into two molecular phenotypes: those with high type 2 T-helper cell expression (T2), and those with low expression (non-T2). T2 asthma patients have multiple targeted biologic treatment options, but these are expensive and around 30-35% of patients do not respond to them. These patients must rely on conventional drugs for symptomatic relief (such as bronchodilators and inhaled corticosteroids), which do little to control severe disease or combat the underlying disease pathology.
To better understand the difference between T2 and non-T2, we performed a comparative study using a UK Biobank dataset with 7,094 T2 asthma patients and 15,071 non-T2 patients. The study identified clear differences in the genetic makeup and disease associated metabolic pathways between the T2 allergic and non-T2 asthma cohorts.
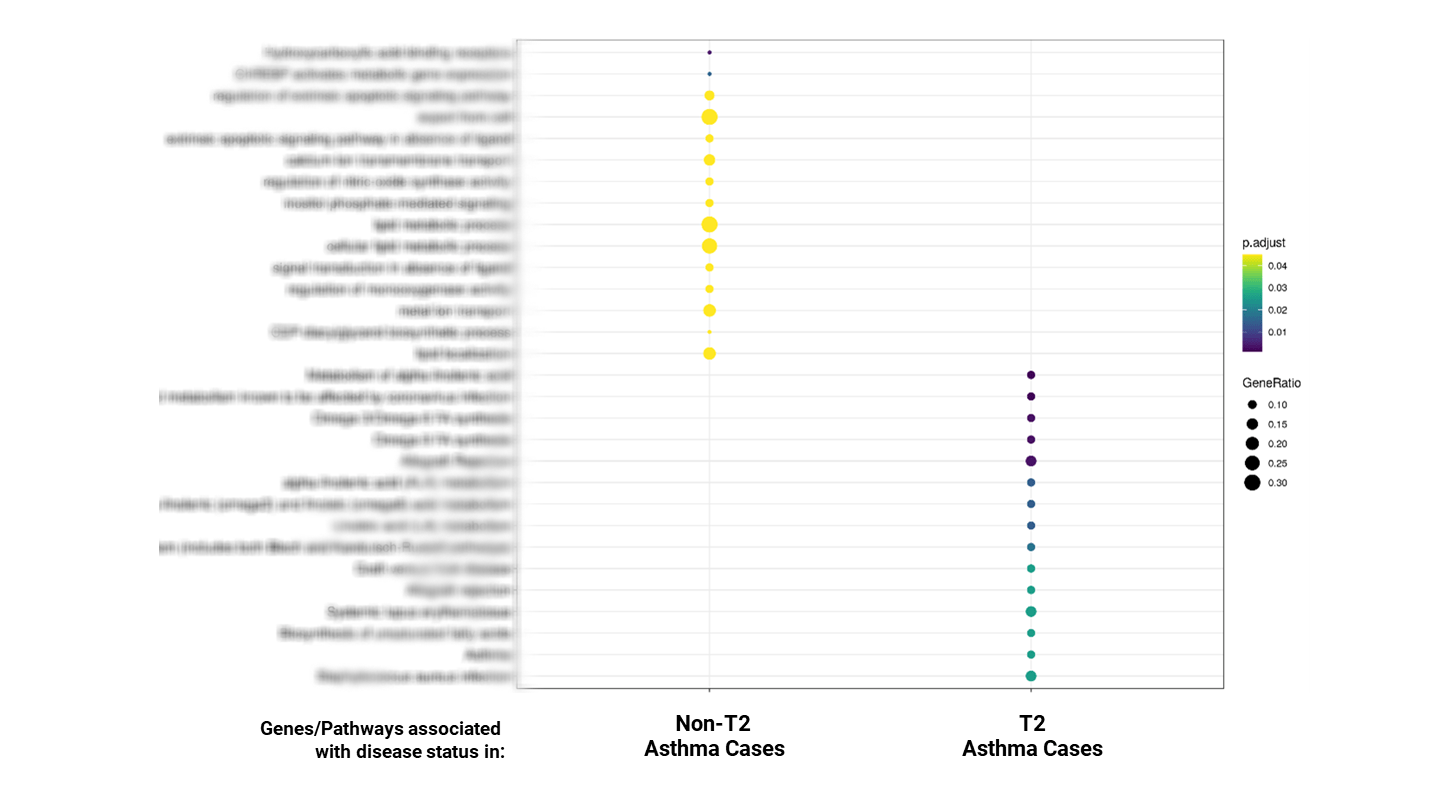
Figure 14. Pathway enrichment results for T2 vs. non-T2 asthma.
These findings hold significant potential for better patient stratification, diagnosis biomarkers, and new treatment options. They clearly demonstrate the differing biological drivers of T2 and non-T2 asthma, opening new options for better diagnostics and therapy section, as well as novel drug targets and therapies that can address the unmet medical need of non-T2 patients.
10. Achieving End-to-end Precision Medicine
The tools that we are developing have the potential to transform understanding of complex chronic diseases that have so far been intractable, and to offer new therapeutic approaches and opportunities for helping patients sooner by making repositioned medicines targeted to them available more quickly than developing drugs from scratch.
This is not just an exercise in target or systems biology, or novel drug discovery, but also in the accurate prediction of risk and selection of the most effective intervention strategy for patients to prevent disease progression.
Ultimately the full benefits of precision medicine will be realized when all of these developments come together – when diseases are diagnosed not by clinical label, but by presence of robust diagnostic biomarkers, when a wide range of therapies targeting different mechanisms are available, whether developed de novo or repositioned, and of course when prevention of disease is as valued as highly as its treatment.
References
[1] Tam V, Patel N, Turcotte M, Bossé Y, Paré G, Meyre D. Benefits and limitations of genome-wide association studies. Nat Rev Genet. 2019 Aug;20(8):467-484. doi: 10.1038/s41576-019-0127-1
[2] Abell NS, DeGorter MK, Gloudemans MJ, Greenwald E, Smith KS, He Z, Montgomery SB. Multiple causal variants underlie genetic associations in humans. Science. 2022 375(6586):1247-1254. doi: 10.1126/science.abj5117.
[3] Rahit KMTH, Tarailo-Graovac M. Genetic Modifiers and Rare Mendelian Disease. Genes (Basel). 2020;11(3):239. Published 2020 Feb 25. doi:10.3390/genes11030239
[4] Mars et al., Genome-wide risk prediction of common diseases across ancestries in one million people (2022), Cell Genomics 2, 100118 https://doi.org/10.1016/j.xgen.2022.100118
Precision Medicine R&D for Chronic Disease
The era of precision medicine is transforming drug discovery and development. But to achieve precision medicine for more prevalent, expensive, and complex, chronic conditions we need a radically improved approach to R&D.
This guide presents a new view of disease biology and an approach to enable biopharma to deliver better, more personalized treatments for unmet medical needs.
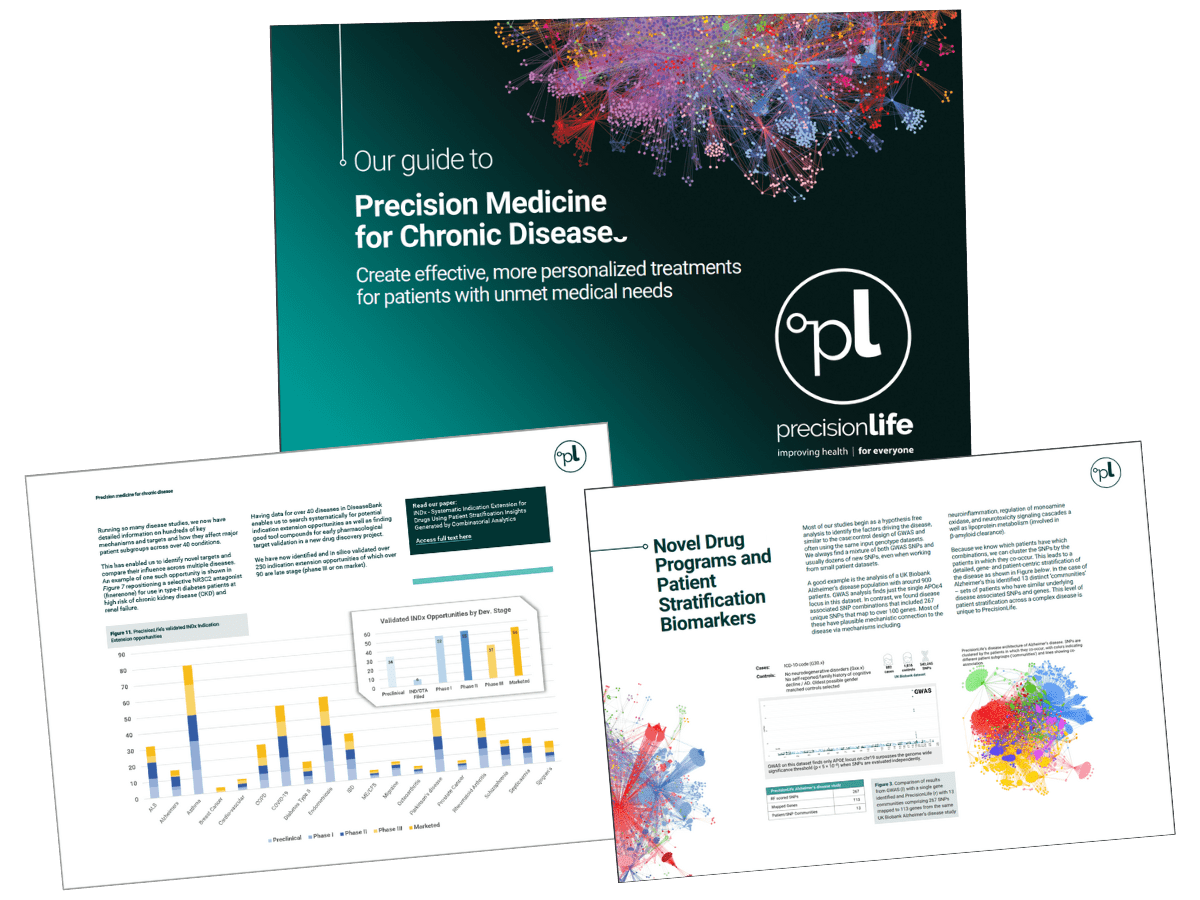