How can mechanistic patient stratification inform R&D in complex chronic diseases?
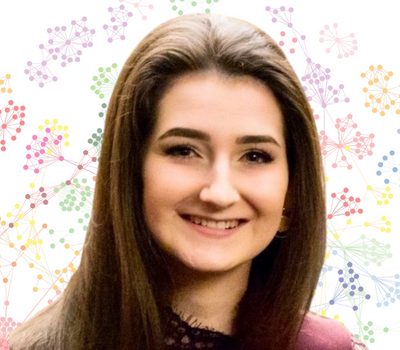
Senior Portfolio Manager
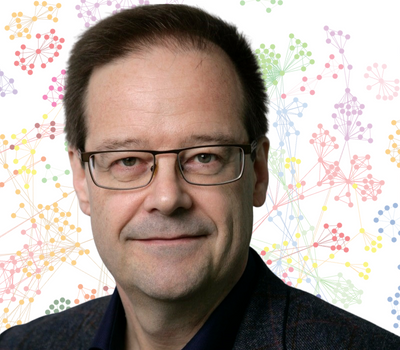
CEO & Co-founder
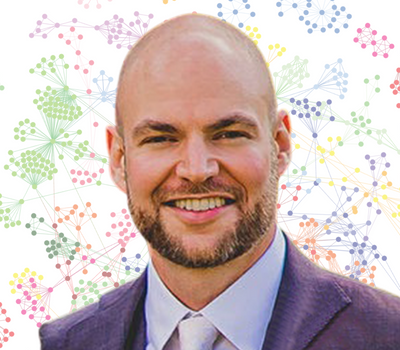
Marketing Director
Groundbreaking disease biology and drug discovery insights revealed by stratifying ME/CFS patients using combinatorial analytics
Patients with a chronic disease may share the same diagnosis, but not necessarily the same mechanistic causes of their disease. So, treatments that are effective for one patient may well not be effective for another.
Because chronic diseases are multi-factorial – usually polygenic and with significant environmental and epidemiological components – they can be incredibly difficult to understand, diagnose and to develop effective therapies to treat them.
This is the case with myalgic encephalomyelitis and chronic fatigue syndrome (ME/CFS).
ME/CFS is a debilitating chronic disease which has 100% unmet medical need with no reliable diagnostic tests or approved disease modifying therapies for the estimated 20 million people living with the condition.
After decades of study, the genetic contributions to ME/CFS and the different mechanisms underpinning the disease remained poorly understood. Previous Genome-Wide Association Studies (GWAS) have aimed to identify significant genetic factors underlying ME/CFS disease risk, but these studies have all been unsuccessful.
GWAS look for common types of genetic variation (single nucleotide polymorphisms or ‘SNPs’) that occur more in a patient population than in a control population, assessing each gene, one at a time, in isolation.
But what happens if genes in complex diseases interact, and their combined interactions produce an effect that is causing or contributing to the disease? Standard analysis, like GWAS, wouldn’t be able to detect these combinations of interacting genes or measure their effect.
That’s where PrecisionLife is fundamentally different. We’ve developed new ways of looking at disease biology at an unprecedented level of resolution, to make patient stratification possible for even the most complex heterogeneous diseases.
With this level of insight, we're breaking new ground in the discovery and development of better, more personalized treatments for chronic diseases.
High resolution patient stratification is key to precision medicine
By capturing the non-linear effects of genetic and metabolic interactions, our combinatorial approach reveals many more of the biological drivers of disease. Combinatorial analytics generates much more signal from much smaller datasets than GWAS, and can incorporate a wide range of multi-modal data, including:
- Genomic data
- Transcriptomic data
- Clinical data
- Environmental data
- Epidemiological data
We analyze the huge number of possible combinations within patient data to identify disease signatures - interactions of features that together contribute to a disease in subgroups of patients.
Figure 1: Combinatorial disease signatures (left) are combinations of SNPs that together are strongly associated with disease and reveal unique high-resolution disease insights.
Once identified, we merge the results of many signatures and cluster by patient co-occurrence to generate disease architectures showing the SNP communities associated with patient subgroups (right).
Combinatorial analytics allows us to understand complex disease biology and stratify patients according to the etiology of their disease, creating mechanistic patient stratification biomarkers.
This means that within a population of patients diagnosed with the same disease, we can identify within that population the subgroups of people who share the same biological drivers underpinning their specific form of the disease and understand which targets and therapies will work for them.
We’re using these insights to:
- Discover better drug targets for treating disease
- Develop new tools to improve diagnosis
- Inform the selection of the most effective medicines for individual patients.
Discovering the first genetic insights of a complex chronic disease
ME/CFS is a severe and severely neglected disease. It has no effective treatment, no diagnostic markers, and no known causes or genetic risk factors.
We applied both GWAS and the PrecisionLife combinatorial analytics platform to analyze ME/CFS cohorts from UK Biobank, including the Pain Questionnaire cohort, in a case–control design with 1000 cycles of fully random permutation (Figure 2).
Our aim was to identify combinations of SNPs that when observed together were strongly associated with the development of ME/CFS.
The standard GWAS analysis of the dataset revealed no significant genes associated with the disease.
We then performed a combinatorial analysis on the same data, and the results were groundbreaking.
84 disease signatures were identified containing combinations of 199 SNPs that together are strongly associated with an ME/CFS diagnosis, including:
- 25 critical SNPs that map to...
- 14 novel genes, that were significantly associated with...
- 91% of the patients in the ME/CFS population.
Figure 2: Overview of the Combinatorial Analysis study of the UK Biobank ME/CFS ‘Pain Questionnaire’ Dataset.
Patient stratification biomarkers for ME/CFS
The analysis produced the first high resolution patient stratification insights for ME/CFS.
Our disease signatures mapped to 15 distinct patient subgroups within the population (Figure 3). Each of these subgroups have shared disease etiologies and are therefore likely to share phenotypes, including severity, progression rate, clinical presentation, and therapy response.
Figure 3: Disease architecture diagram demonstrating the 15 patient subgroups. Left: each circle represents a disease-associated SNP, lines represent their co-association in patients in disease signature(s), and colors represent distinct patient subgroups. Right: the same disease architecture highlighting 25 critical SNPs associated with the subgroups.
By focusing on a particular subgroup, we can develop a mechanism of action hypothesis and understand which targets and drugs will work for specific patients.
An example of this can be seen in Subgroup 9 (Figure 4) from the disease architecture.
The critical SNP in is this subgroup (rs3785477), was mapped to gene AKAP1, which regulates mitochondrial respiration. This is likely involved in post-exercise recovery and would explain impaired recovery and severe fatigue often experienced by people living with ME/CFS.
This subgroup includes 648 (27%) of the 2,382 ME/CFS patient cases we analyzed, meaning over a quarter of patients would benefit from treatments targeting its mechanisms.
Figure 4: Summary of patient stratification results from ME/CFS Subgroup 9 with the mapped gene AKAP1 in the PrecisionLife study.
“With these findings we can develop mechanism of action hypotheses and patient stratification biomarkers to guide future diagnostics and drug discovery programs for precision medicines targeting specific patients, for example, those in the prevalent Subgroup 9, which includes over a quarter of the patient population we analyzed.”
Krystyna Taylor, co-author of the ME/CFS study and Senior Portfolio Manager at PrecisionLife.
Let's compare with findings from another subgroup.
In Subgroup 15 (Figure 5) the critical SNP (rs2304725), was mapped to gene SLC6A11, which encodes GAT3, which is a GABA re-uptake transporter. Altered GAT3 levels are associated with cognitive impairment, vulnerability to stress, and sleep disturbance - all of which can be experienced by ME/CFS patients.
By reviewing the clinical phenotypes of this subgroup, we found that cases have significant association with increased phenylalanine levels, which is a precursor to monoamine neurotransmitters such as dopamine and serotonin. This suggests that patients in subgroup 15 are more likely to experience some of the neurocognitive effects of ME/CFS.
Figure 5: Summary of patient stratification results from ME/CFS Subgroup 15 with the mapped gene SLC6A11 in the PrecisionLife study.
Replicating the results
To investigate the extent to which the results generated from the original Pain Questionnaire cohort could be replicated in these cohorts, we tested for replication of the 25 critical SNPs in three additional disjoint cohorts of patients (Verbal Interview CFS, post-viral syndrome and fibromyalgia). Overall, 7 of the critical SNPs exhibited significant association with disease in at least one other cohort, with 2 replicated across multiple cohorts.
We aim to replicate and extend the results from this UK Biobank study with combinatorial analysis of a future DecodeME study. DecodeME is the largest current genetic ME/CFS study, with over 20,000 participants involved, and the more detailed patient survey data collected is likely to allow deeper insights into the different subgroups and targets involved with the disease.
Cross-disease precision medicine insights
Comparing our ME/CFS findings across other diseases in our DiseaseBank revealed interesting similarities with other diseases and post-viral syndromes.
Multiple sclerosis (MS) and ME/CFS patients share several similar symptoms, including pain, sleep disturbance and cognitive dysfunction. There is also increasing evidence that many patients diagnosed with long COVID share similar symptoms, such as chronic fatigue and ‘brain fog’, with individuals with ME/CFS. This suggests that the diseases may share similar etiologies with possible overlap in the biological drivers and risk genes.
Our analysis of the first UK Biobank COVID-19 population identified four genes out of 68 associated specifically with the risk of severe COVID that we had previously identified as having strong association with neurodegenerative processes.
We're now in the process of analyzing populations in long COVID-19 and multiple sclerosis to identify any shared genes and biological mechanisms underpinning ME/CFS, multiple sclerosis and long COVID-19. Preliminary findings have indicated that three of the genes we identified in our ME/CFS study are also significant in the long COVID patient group.
By uncovering the disease etiologies, providing better patient stratification, and identifying novel drug targets in ME/CFS and disorders with similar symptoms we aim to accelerate progress in the approval of better diagnostic tools and effective treatment options for patients.
The full write-up of the study is available to read in our open-access paper, Genetic Risk Factors for ME/CFS Identified Using Combinatorial Analysis, published in the Journal of Translational Medicine.
Where can this patient stratification take us?
There are currently no specific pharmacological treatment options for ME/CFS patients or many other groups of patients with a chronic disease.
This ME/CFS disease study represents the first time that replicable genetic findings have been reported in over 30 years of research into the disease, offering new opportunities for precision medicine.
With our patient stratification biomarkers, it is now possible to accurately identify all the clinically-relevant patient subgroups in ME/CFS to inform drug discovery programs, future clinical trials, diagnosis and prescription of effective treatments - improving the probability of successful drug development and market access.
Most immediately, having performed combinatorial analysis in over 40 complex chronic diseases, we’re able to systematically map the target biology of clinically relevant patient subgroups across diseases to identify secondary indication potential for existing therapies and late-stage drug candidates. This has led us to investigate three potential drug repurposing opportunities that might be beneficial to subgroups of ME/CFS patients.
Derisking biopharma innovation in chronic diseases
We’ve developed an extensive pipeline novel drug targets and precision drug repositioning opportunities across multiple diseases and therapeutic areas, each supported with patient stratification biomarkers, explanatory mechanism of action, and genetic linkage.
With our patient stratification insights increasing the likelihood of downstream success, these assets represent valuable opportunities for biopharma to derisk and optimize every stage of drug discovery and clinical development – addressing the unmet medical needs of patients in some of the most challenging and most common chronic diseases.
Get in touch with us to discuss partnering opportunities
Send us a request using to the contact form below or click on the button to find out more about partnering and collaboration opportunities with PrecisionLife.
Contact us
Ask us a question or contact us to discuss collaborations and partnership opportunities, by sending us a message - we'll get back to you as soon as we can.
Sign Up