Fixing drug discovery & development for chronic diseases
The blockbuster model for drug discovery and development in chronic diseases is inefficient and costly.
We need a new approach that puts patients and their diverse disease biology at the centre of the R&D process.
The one-size-fits-all model of medicine is broken
The traditional approach to discovery, development and delivery of medicine is bad for everyone in the ecosystem. It’s bad for patients, who are often getting drugs that don’t work for them, with many of even the most prescribed medicines being effective only for a minority of patients. It’s bad for healthcare systems, prescribing expensive but ineffective medicines and having to care for patients whose underlying medical needs are not being served. And it’s bad for pharma too.
Productivity in drug discovery research and development is decreasing, with R&D costs rising in part due to failures for new treatments in late-stage clinical trials and pressure on forecast sales due to increasing unwillingness to pay for medicines that can’t be demonstrated to have the desired outcomes in patients.
Pharma needs better understanding of disease biology and better drug targets that will be successful in clinical trials; healthcare needs to reduce ineffective prescriptions; and patients need to be diagnosed accurately and prescribed drugs that will work for them.
Developing effective drug treatments is never easy
Precision medicine is the way forward, but it’s an incredibly complex challenge for pharma to develop more personalized treatments, especially in some of the areas of largest unmet medical need.
Pharma productivity and the return on investment of R&D have reached record lows. Late-stage failures in phase III, where treatments are failing to demonstrate efficacy, are particularly costly and disruptive.
Efficacy requires knowing which patients will benefit from a treatment in order to recruit smaller, more focused clinical trial populations. This requires greater insight into the disease biology and how those targets map to patient subgroups. For example, Alzheimer’s disease drug trials have had a 99% failure rate over the last decade.
This is not because anyone gave less than 100% trying to find a better therapy, but because the patients on whom the molecules were being tested were not effectively selected using stratification biomarkers to identify those most likely to respond positively to the treatment.
This requires deeper insights into the disease biology than just noting single genetic markers or clinical features.
Not being able to identify the patients who are going to benefit from a medicine also has consequences for the rate of clinical adoption. Even in an area of high unmet medical need, defending a high price point for drugs without being able to predict patients for whom they will be effective is unrealistic in an era of pressured healthcare spending.
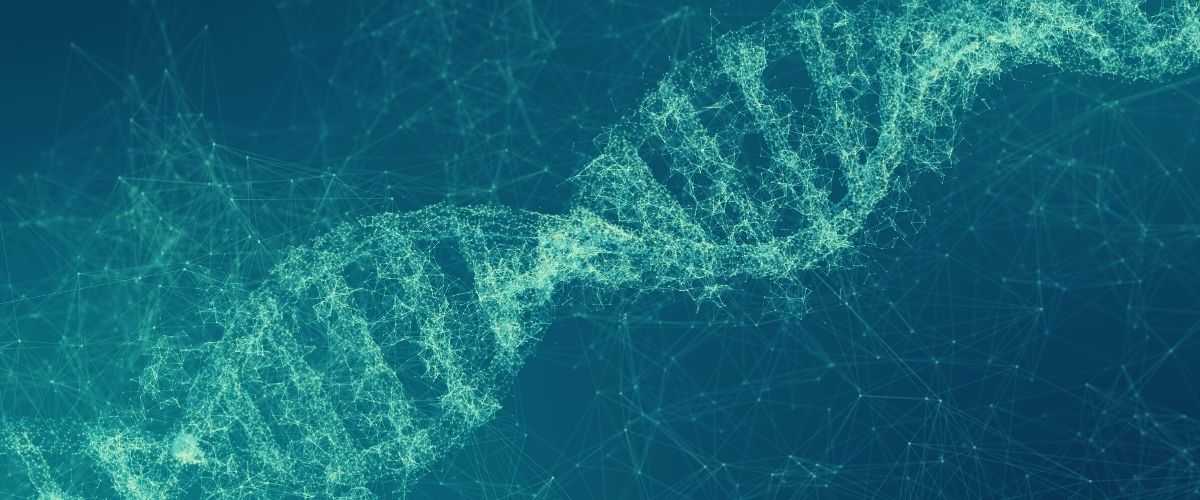
Why hasn’t genomic analysis solved the problem?
The hope was that with the announcement of the draft Human Genome Project in 2000 a lot of these complex challenges would be resolved. The thinking was that we would find mutations associated with many diseases and be able to design drugs relatively quickly to solve them.
Tools such as genome wide association studies (GWAS), are used to analyze large scale patient genomic data, to locate significant single mutations that occur regularly in many disease cases and very rarely in controls. This has worked in cancers driven by somatic mutations and rare genetic diseases, where major advances have been made.
Unfortunately, mutations and other factors which are less frequent, affecting smaller groups of patients won’t be detected. Nor will combinations of relatively common variants that interact in more complex ways to create an unexpectedly large effect on a disease phenotype.
So, if the biology of a disease includes something that’s relevant only to a certain subgroup of a patient population, or that occurs when the effects of more than one mutation come together, you’re unlikely to find it using standard genome wide association studies.
Chronic diseases are not caused by a single genetic change, they are caused by multiple interactions in combination.
They often present as a spectrum of symptoms, and patients with the same diagnosis may have different underlying causes of a disease. This can further confuse GWAS analysis, which works at a study population level and cannot differentiate the varied disease factors at work in different subgroups of patients.
This is the challenge that has limited what researchers have been able to achieve with genomic analysis of patient data in complex, chronic diseases over the last 20 years.
How can we personalize chronic disease drug discovery and development?
Chronic diseases such as cardiovascular disease, dementia and diabetes exert a huge socioeconomic cost, and a major, growing disease burden for patients. They kill over 40 million people a year, making up seven out of ten deaths globally. Chronic diseases are the leading causes of death and disability in the United States, accounting for 80% of all healthcare costs.
Addressing the urgent medical need in chronic diseases by identifying risks early, developing effective treatments, and getting them to the right patients is incredibly challenging because chronic diseases don’t work the same way as monogenic diseases.
Chronic diseases are multifactorial, where the biology that drives them contains feedback loops, some of which inhibit each other, and some of which amplify each other’s effects. These generate complex interactions of genetic and metabolic networks, with unpredictable, non-linear results that are impossible to predict using the outputs of GWAS analysis.
Chronic disease population datasets diagnosed by clinical observation also usually contain overlapping sets of different patient subgroups. These may share a clinical diagnosis but the causes of disease, severity, progression rates, symptoms and treatment response may well be different in each of these subgroups.
This creates a real challenge in chronic disease drug discovery and development but also a real opportunity for novel target discovery.
Combinatorial analytics: an essential tool for the delivery of precision medicine
The traditional one-size-fits-all model of medicine is broken. If we are to address the global burden of chronic disease so that people can live longer, healthier lives, we need to improve our understanding of complex diseases and create better, more personalized therapy options.
We need a precision medicine approach to chronic disease drug discovery and development with a whole new way of looking at chronic disease biology, analyzing large-scale patient populations to detect signal in complex biology and finding biomarkers to identify patient subgroups, and the mechanisms of action and targets that will be effective for those patients – taking drug development and prescription from population to personal.
Achieving precision medicine in chronic diseases requires the ability to look at combinations of features and how they associate with disease subgroups, which is only possible with combinatorial analytics.
Speak to PrecisionLife to find out how to apply this unique approach to your R&D programs for:
- Novel target discovery
- Patient stratification biomarkers
- Label extensions
- Optimized clinical trial design
- Creating value from parked assets
Watch the webinar on this topic to learn more
Steve Gardner, CEO of PrecisionLife joined Naheed Kurji, President & CEO at Cyclica to discuss the challenges of target discovery and how using a combinatorial analytics approach can overcome them to improve chronic disease drug discovery and development outcomes.
The webinar is available to watch on-demand now.
Contact us
Ask us a question or contact us to discuss potential collaborations and partnership opportunities, by sending us a message here and we'll get back to you as soon as we can.
Form header
Sign UP